AI in QA?
- Les Rowe
- Feb 22, 2024
- 3 min read
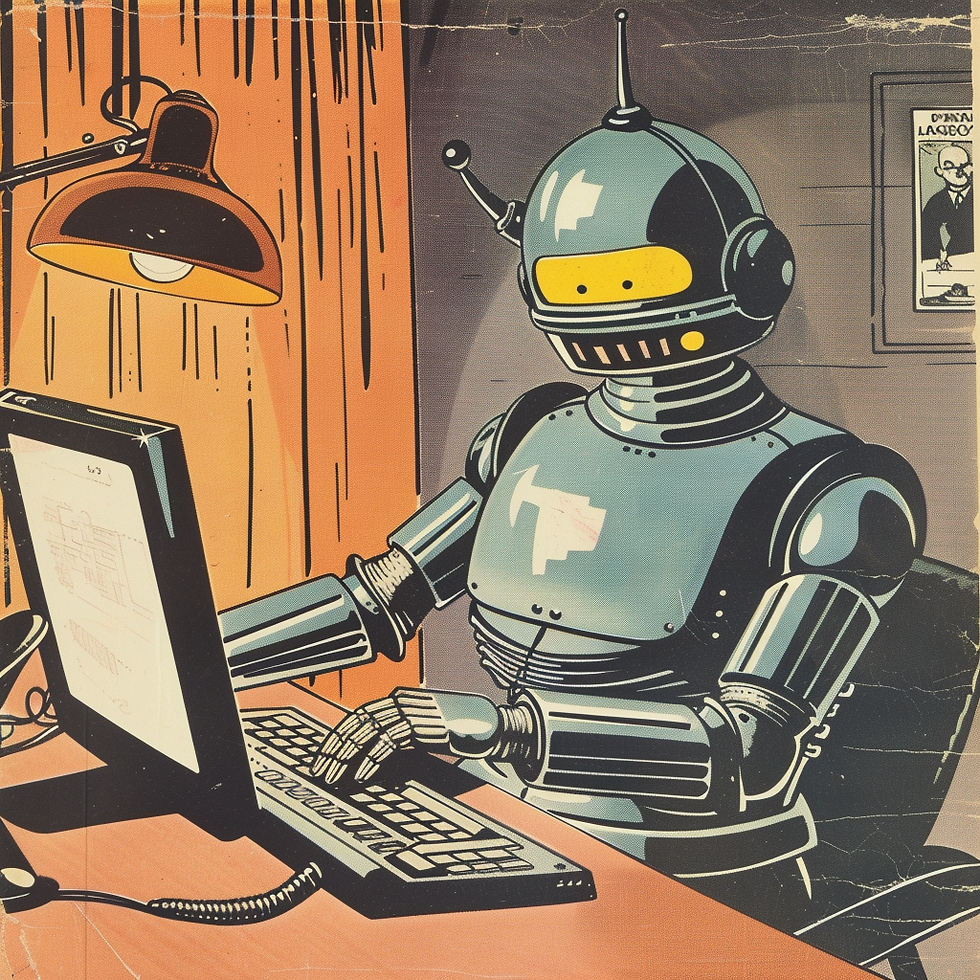
In an era where digital innovation is at the forefront, Artificial Intelligence (AI) has emerged as a pivotal force, revolutionizing industries far and wide. The realm of software development, with its ever-evolving complexity and demand for perfection, is no exception. As we delve into the intricacies of software Quality Assurance (QA), a domain traditionally reliant on meticulous human effort, the integration of AI stands out as a beacon of progress, promising to redefine the landscape of software testing.
Why AI in QA?
At the heart of software development lies the crucial phase of QA, where developers and testers toil to uncover bugs and ensure that the software aligns seamlessly with its intended requirements and user stories. This process, while fundamental, is often time-consuming and prone to human error. Enter AI, a game-changer that brings with it the allure of automation, precision, and efficiency. By leveraging technologies such as Machine Learning (ML) and Natural Language Processing (NLP), AI transforms the QA process into a more streamlined and effective operation.
The Benefits Unveiled
The incorporation of AI into QA processes unveils a plethora of benefits, paramount among them being the automated generation of test cases from requirements and user stories. This not only accelerates the testing cycle but also ensures a broader coverage of test scenarios, reducing the likelihood of oversights. AI's ability to sift through and analyze vast datasets allows for the identification of patterns and anomalies, enhancing the accuracy of bug detection. Furthermore, AI-driven testing tools continuously learn and evolve, adapting to new challenges and refining their algorithms for better outcomes. Perhaps most impressively, AI can prioritize test cases based on historical data, focusing efforts on areas most likely to harbor defects.
Navigating the Challenges
Despite its promising advantages, the integration of AI into QA is not without its hurdles. The effectiveness of AI-driven testing hinges on the availability of high-quality, relevant data to train the models, a requirement that can pose significant challenges. Additionally, the implementation of AI solutions necessitates a delicate balance between automation and human oversight. While AI excels at identifying clear-cut bugs and patterns, nuanced issues may still require the discerning eye of a human tester. Moreover, the initial complexity and cost of integrating AI into existing QA workflows can be daunting for organizations, especially those with limited resources.
Real-world Impact and Future Prospects
The transformative impact of AI on QA is not merely theoretical. Companies across the globe are increasingly harnessing AI to streamline their QA processes, with notable success stories underscoring the technology's potential. Tools and platforms that leverage AI for test case generation, bug tracking, and predictive analytics are gaining traction, offering a glimpse into a future where AI-driven QA is the norm rather than the exception.
As we look ahead, the trajectory of AI in QA seems poised for further innovation. The potential for more sophisticated predictive analytics, deeper integration with development processes, and enhanced automation capabilities promises to elevate software testing to new heights. For organizations and individuals alike, staying informed and adaptable in the face of these advancements will be key to harnessing the full potential of AI in QA.
Conclusion
The integration of AI into the software QA process represents a significant leap towards more efficient, accurate, and effective testing methodologies. While challenges remain, the benefits of embracing AI in this domain are undeniable, offering a pathway to improved software quality and development efficiency. As we venture further into this digital age, the role of AI in QA will undoubtedly continue to evolve, shaping the future of software testing in ways we are only beginning to imagine.
Comments